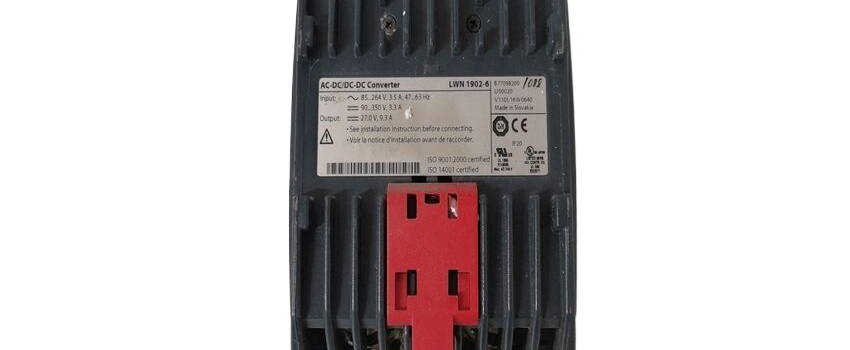
Vision AI enabled the automation of many complex, monotonous and dangerous tasks, but the reliability of most vision AI powered robotic cells has long disappointed. Early iterations provided lackluster performance below a reasonable usability threshold, with excessive error rates. The introduction of supervised machine learning to vision AI improved performance, but most cells relying on this technology still make erroneous picks approximately six out of every 100 tries when handling tasks like parcel induction or order fulfillment. Even the world’s largest e-commerce marketplaces still fail more excessively when picking transparent poly bags and handling other highly complex tasks.
While some early adopters learned to live with these shortcomings, others waited for more acceptable performance, suppressing industry adoption of vision AI for variable piece picking. Cascade learning has changed the game, attaining long-desired reliability with near 100% success rates in item picking.
Vision AI helped automation; room for improvement remains
Cluttered environments and variance-filled tasks require robots that can see and process quickly and accurately; vision AI touts many well-established examples of complex tasks that can now be automated:
- Item/order picking in e-commerce, apparel and groceries has been partially automated for nearly a decade.
- Mixed-SKU (rainbow) depalletizing, including even the most challenging tasks of depalletizing mixed heavy bags and boxes weighing up to 100 pounds (45 kilograms).
- Shippers, transportation companies, distribution centers and others leverage vision AI for more organized stacking to reduce air space during shipment or storage.
At the bleeding edge of this innovation, loose loads trailer and container unloading represents the next frontier. Loose loads offer supply chain and logistics pros a greener solution that can reduce carbon emissions since loose loads can fill trailers up to 96% versus palletized loads that can only fill trailers approximately 60%.
However, older robotic cells often rely on traditional computer vision and top out at 70-80% success rates. Newer installations that integrated supervised machine learning still struggle with unacceptable error rates despite improvements.
This leads to goals only being partially achieved. The goal, for example, might be for 10 robots to complete 10 jobs independently, but far more often, these 10 robots will require a few human supervisors to intervene and deal with miss-picked and double-picked items. In each case, automation has fallen short of its true potential.